Cardiometabolic Health Congress (CMHC) asked Eric D. Peterson, MD, MPH, for his expert opinion on how artificial intelligence (AI) is impacting health care and how it will shape the future for both patients and providers.
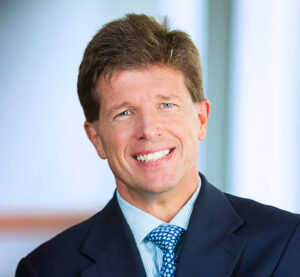
Eric D. Peterson, MD, MPH, is a professor of Internal Medicine and faculty of the Cardiology Division at the University of Texas Southwestern Medical Center, where he is the Adelyn and Edmund M. Hoffman Distinguished Chair in Medical Science. He earned his medical degree from the School of Medicine at the University of Pittsburgh and completed a Master of Public Health from Harvard School of Public Health. He completed residency training at Brigham and Women's Hospital and fellowships at Harvard University School of Medicine and Duke University Medical Center. He has published extensively on vital cardiology topics and has a special interest in the use of AI in the advancement of the specialty.
CMHC: A potential role of AI in health care is to act as a watchdog for the medical errors that cost an estimated $1.9 billion and more than 200,000 lives annually. How can AI models address these costly oversights?
Dr. Peterson: I think AI serves the potential to reach that goal, which was actually raised by another technology years ago; we thought the electronic health record (EHR) was going to fix a lot of those problems. We found out it was a decision-support tool effective for billing, but that it wasn’t actually very effective at reducing medical errors.
A simple example of how AI can do this: You have a penicillin allergy that was missed in your last EHR note. I look at this last record and don’t see a contraindication, so I order penicillin for you. AI could give me an alert to prevent a potentially serious reaction before the prescription is filled. A more complex example: You have heart failure with reduced injection fraction but your physician isn’t aware of a new evidence-based medicine that would be appropriate for you. AI could look through your health data and recommend these newest therapies based on all the evidence it has been trained on.
"As we move forward AI will become a helpful decision-support tool for doctors to reduce medical errors."
CMHC: Is there a limitation of using AI to predict health outcomes if the model is trained on existing medical data that may be biased?
Dr. Peterson: There are several aspects to this, the first of which deals with complex AI models being ‘overfit.’ AI models develop complex predictions based on multiple pieces of data. Say they predict a patient’s likelihood of developing a severe infection called sepsis. If that model is developed on a limited number of cases or highly selected patients, then the prediction model may be biased and may not accurately measure results in a general population. For example, say we develop an AI model in a single hospital system and we find out that by chance patients named Bob had higher likelihood for sepsis than others. An unsupervised AI model might use these data to predict that future patients named Bob also would have a higher risk. As a clinician, you’d know that a person’s name alone doesn’t truly predict risk, but these AI models often are opaque, and it can be difficult to know what predictors they use.
A second issue is, say we develop a model in the setting of academia where care is practiced in a certain pattern, but then we apply it to a community where practice patterns, patients, and other factors are very different. That model may perform very differently in that new population and may under- or over-predict outcomes.
A third example is that models may be biased against certain patient subgroups. Let’s imagine that African American patients are under-treated for a condition and thus are more likely to die. An AI predictive model could include race as a predictor of higher risk and this high-risk prediction could guide doctors to inappropriately treat black patients in the future.
"If the sample used to develop an AI model isn't very robust, it could result in being 'overfit' – its predictions fit too closely to the training dataset and aren't generalizable to other populations or settings."
Dr. Peterson: A final issue is that even well-developed and highly predictive models may actually change over time. We put these models in place and, because they are validated and perform well in our system, we assume they will perform well. However those models tend to change their predictive accuracy as practice or patient populations change, so they need to be monitored to make sure they are performing well over time.
CMHC: How can providers spot these AI models that may be based on skewed data to avoid relying on inaccurate predictions?
Dr. Peterson: All four of the examples of biases I gave exist, and consumers of AI models need to be on the lookout for them. There are several things you’re going to ask:
- Is the model validated?
- Are the variables that go into the model things that I as a clinician would think are logical predictors?
- Does the model perform consistently and predictably over time?
- Does the training data reflect the population and setting in which the model is being used?
“In medicine, we as physicians all the time make errors or miss opportunities to provide evidence-based treatments and we accept that as standard of care. Now, say occasionally a machine might make an algorithm-based suggestion and get it wrong. That's probably still better than we did.”
Dr. Peterson: As a clinician, you should ask how a prediction model performs in populations like yours. That’s simply having your hospital or health system test to make sure that a model is actually accurate in your system. Also, it is important to check a model over time to revalidate its accuracy as a function of time. Finally, it’s important to be sure the model isn’t biased by factors such as race, gender, age and other characteristics that shouldn’t affect care decisions.
CMHC: Are there plans for a regulatory body focused on the use of AI in health care?
Dr. Peterson: The biggest concern for the FDA with regard to AI regulation surrounds ‘black box’ AI, which is when a clinician can’t clearly see or validate the elements a given AI algorithm uses for its logic. In that case, the FDA requires close review and regulatory approval before its use in clinical practice. But, given the speed of AI model development, you can see this has created a huge backlog of models that need to be reviewed by the FDA. On one hand, society wants to speed AI innovation and use to improve outcomes for patients, so we don’t want to delay that innovation for too long. On the other hand, because there could be harm to patient care, these models require some regulation. Finding the right balance between speed and safety is a major challenge for the FDA.
“There is a gray line of ‘what is software as a medical device?’ that has come from the U.S. Food and Drug Administration (FDA) which determines whether AI models need to be regulated."
Dr. Peterson: If a clinical decision is actually overseen by a physician it creates less trouble because the assumption is that it will be given the same consideration as any other clinical decision. Much stronger regulatory support would be needed over things like saying I’m going to change your insulin pump or I’m going to change your medication without a physician’s oversight. Once you give the machine the power to treat, then it’s clearly a different level of oversight needed than if it’s just saying “You should consider a statin for this patient.”
CMHC: What do you say to providers concerned that AI will eliminate jobs or result in Hollywood movie-type mayhem?
Dr. Peterson: These technologies are designed to be used to aid provider decision-making and, until a governing body exists, clinicians must rely on the guidelines in their field and should never use an AI suggestion to counteract guideline-directed care. If a model came up with an odd prediction because of some wrongly abstracted number, you as a physician are still able to say, “That doesn’t make sense, I’m not going to act on that prediction in this case.” We do this in lots of areas. We take blood pressure readings and if we see one that seems way off we’ll repeat it. That’s a part of what we’re used to in medicine and we’ll continue to control that button.
“Occasionally, self-driving cars have failed in practice and people have been accidentally hit. While that's true and horrific for the individuals involved, one has to remember the rate of accidents caused by human drivers is much higher than that seen with these computer-driven machines. We don't seem to have any trouble with the fact that humans hit people with cars all the time, but we don’t tolerate any mistakes by machine-driven ones."
Dr. Peterson: Also, this idea of AI in medicine isn’t new. When you get an EKG done in this country, they all have an interpretation at the top based on a machine. These machine algorithms are based on review of millions of past EKGs. Over time, these EKGs have become quite accurate at indicating when a patient has conditions such as a possible acute myocardial infarction. I’m a cardiologist and I have to admit that these machine interpretations sometimes help me to consider some condition I might have missed without a prompt. These make me a better doctor.
“I think physicians' fears of AI, to the most extent, are greater than they need to be. Most of the AI that we're talking about in the near term are things that will facilitate our practices, not replace us.”